’Identifying potential fraud at quote stage could potentially save the industry millions of pounds,’ says chief executive
Machine learning and artificial intelligence specialist MLP has released a new fraud prediction model for the insurance market.
Called Propensity to Defraud, the modelling tool helps firms carry out a risk assessment on personal lines applicants at the point of purchase.
It will then say how likely someone is to commit fraud, assigning them a probability score from 1% to 99%.
The tool is able to do this by drawing in data from multiple sources – this is then overlayed with knowledge and experience from fraud experts.
MLP chief executive Damian Baxter said: “Identifying potential fraud at quote stage could potentially save the industry millions of pounds and free-up budgets to support innovation, customer care and risk management.
“As intermediaries, brokers are at the vanguard of preventing fraudsters from getting into the insurance system. Those able to block them early, quickly and reliably will be recognised by insurer partners.”
Data
MLP, which is part of Open GI, said it launched the tool after the ABI reported that insurers detected 72,600 dishonest insurance claims in 2022, valued at £1.1bn.
Read: Axa defeats Google-spoofing advertiser
Read: Gym buff out-muscled by Markerstudy after dishonest claim
Explore more fraud-related articles here or discover more news content here
“Fraud is an enormous problem for the insurance sector, driving up claims costs, eating into staff time and leaving honest customers losing out as it hikes up premiums,” MLP said.
It developed and tested its new tool in partnership with insurance firm Adrian Flux.
Gerry Bucke, underwriting and sales manager at Adrian Flux, said: “We’ve been delighted by just how effective Propensity to Defraud has been for us. It ensures our teams can focus on helping the business to grow rather than spend time on administrative fraud checking tasks.
“What they are able to do with data and the intelligence their machine learning models bring within milliseconds has strengthened our insurance books.
“Fraud is an industry wide problem and we’re proud to have helped develop what we believe will be an industry wide solution.”
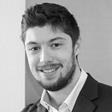
His career began in 2019, when he joined a local north London newspaper after graduating from the University of Sheffield with a first-class honours degree in journalism.
He took up the position of deputy news editor at Insurance Times in March 2023, before being promoted to his current role in May 2024.View full Profile
No comments yet